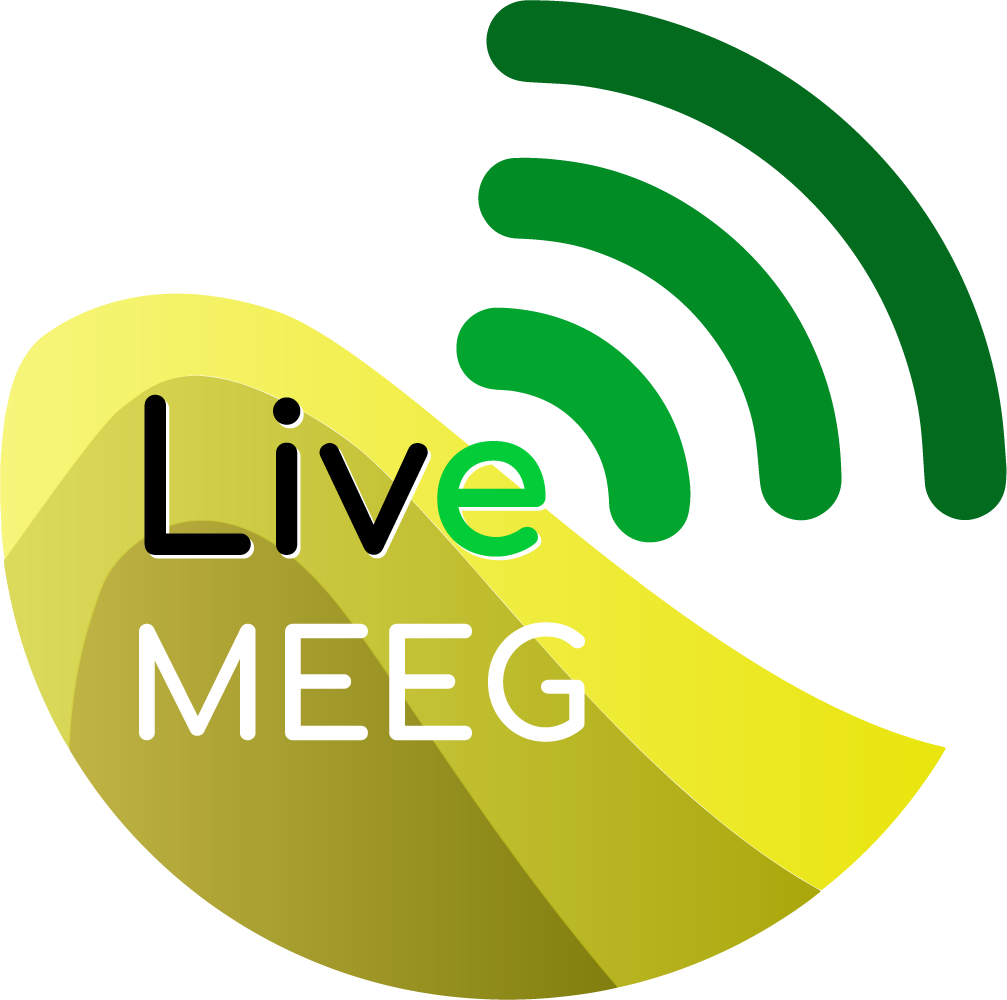
Oct 5-9, 2020
First edition
Virtual event on
Good Scientific Practices in EEG and MEG research
Replay
To view all LiveMEEG recorded content, click here!
Sessions
Good Research Practices: what makes a reliable M/EEG project?
Click here to import all sessions to your Google calendar.
Click on session titles to view the detailed schedule.
Human factors
Know your own, and others’ biases while planning and conducting research – Manuel Mercier, Madison Elliott, Walter Sinnott-Armstrong
Pre-registration
Current and upcoming pre-registration practice in the lab, and in the editorial system – Sophie Herbst, Roni Tibon, Pia Rotshtein
Data Collection
Anticipate to ensure the quality for your data and meet the standards – John Mosher, Katia Lehongre, Giovanni Mento, Emily Kappenmann
Signal processing
Know the strengths and limits of your methods – Karim Jerbi, Jean-Marc Lina, Alex Gramfort
A new Reporting Framework for ERP research
A draft proposal and roundtable discussion (Guest session) – Anđela Šoškić, Suzy Styles, et al.
Reliability
On the reproducibility of M/EEG research – Bertille Somon, Yuri Pavlov, Aina Puce
Collaborative tools
Learn about new tools for open collaboration – Guiomar Niso, Martina G. Vilas, Antonio Schettino
Software (mis)uses
At your own risk: great tools don’t make good practices – Alexandre Gramfort, Scott Makeig, Sylvain Baillet, Robert Oostenveld
Statistics
Power, reliability, and robustness – Maximilien Chaumon, Aaron Caldwell, Steven Luck
Coded tools
Great software tools for improved research practices– Marijn Van Vliet, Laurens Krol, Etienne Combrisson
Beyond the signal
Taking a step back, and thinking about practices in the long run… – Daniele Schon, Yseult Hejja Brichard, David Poeppel
Program
Monday 5 October
Time
Speaker’s name
Talk Link
13:30 (Paris) / 7:30 (NYC)
Human Factors (Chair: Maximilien Chaumon)
14:00 (Paris) / 8:00 (NYC)
Manuel Mercier
The influence of cognitive biases on researchers and scientific production: the inner practice of science for science.
In Wikipedia, a cognitive bias is defined as “a systematic pattern of deviation from rationality in judgment”. As any human being, researchers are prone to cognitive biases, which is a critical matter as these biases can lead to an “unsustainable” science. In this talk, and after a brief introduction on cognitive biases, we will see how they can influence our research for instance through perceptual distortion, illogical choice or misinterpretation. Next, we will envisage some remedies to counteract our inner trend for irrationality. Finally, together with the M.E.EG community we will discuss what can be done at a larger scale to reduce the impact of cognitive biases on scientific practices.
14:20 (Paris) / 8:20 (NYC)
Madison Elliott
What vision science can teach us about best practices in data visualization
It is now easier than ever to visualize experimental data, but today’s super-accessible tools and libraries don’t necessarily help us communicate the meaning of our findings effectively. Fortunately, collaborative work from vision scientists and visualization researchers has yielded some clear design guidelines and heuristics that are based on empirical behavioral results. In this presentation, I will give a brief introduction to design practices and the components of visualization development. I’ll discuss how to put this knowledge to use with your own data through a series of practical examples and “visualization makeovers”. Attendees should leave with a better understanding of viewer tasks and the effectiveness of popular visual encoding choices for EEG and MEG data.
Break
14:50 (Paris) / 8:50 (NYC)
Walter Sinnott-Armstrong
Some common fallacies
All humans make mistakes, and neuroscientists are no exception. We need to understand and watch out for common fallacies in reasoning in order to avoid them in neuroscience research, just as in everyday life. My talk will focus on a few fallacies that are common in some kinds of neuroscience research, including EEG, and illustrate these fallacies with real examples.
15:15 (Paris) / 9:15 (NYC)
Round Table
Break
Pre-Registration (Chair: Antonio Schettino)
16:00 (Paris) / 10:00 (NYC)
Sophie Herbst
Preregistered reports in M/EEG research – a road map through the garden of forking paths?
M/EEG analyses pipelines combine a multitude of processing steps, such as filters, artifact removal, time-frequency transforms, etc., to be chosen by the experimenter. Given that it is impossible to test the independent contribution of each step to the results, novice and even expert neuroscientists are often left with the frustration to not know how strongly a given effect (or the absence thereof) depends on the choices made in their pipeline. Preregistration provides a potential remedy to this problem in that the pre-and post-processing steps are fixed before the study is conducted, and, importantly, expert feedback can be obtained early.
Based on recently obtained ’in-principle-acceptance’ for an EEG replication study, assessing the seminal finding of enhanced delta phase coherence by temporal predictions (Stefanics et al. 2010), I would like to discuss to which extent preregistration can foster replicable and robust EEG/MEG research, and help the community to devise less user-dependent pipelines.
16:20 (Paris) / 10:20 (NYC)
Roni Tibon
Prereg posters: Presenting planned research in academic events
We recently proposed ‘prereg posters’—conference posters that present planned scientific projects—as a new form of preregistration. The presentation of planned research, before data are collected, allows presenters to receive feedback on their hypotheses, design and analyses from their colleagues, which is likely to improve the study. In turn, this can improve more formal preregistration, reducing the chances of subsequent deviation, and/or facilitate submission of the work as a Registered Report. In my talk, I will review data collected at the BNA2019 Festival of Neuroscience, where prereg posters were recently implemented. I will show preliminary evidence for the value of prereg posters in receiving constructive feedback, promoting open science and supporting early-career researchers. I will then discuss the outlook of prereg posters, particularly in the context of the current shift towards online academic events.
Break
16:50 (Paris) / 10:50 (NYC)
Pia Rotshtein
Pre-Registered Reports of Cognitive Neuroscience Research
Pre-Registration is a new format of peer reviewed paper. In conventional/traditional peer review papers authors forms a research question, design a study, collect data, analyse it and write a manuscript that report their research and results – this is then evaluated by peer experts. In contrast, in pre-registration reports (RR), peers evaluate the research before the authors collect the data. Papers gains in principle acceptance, if reviewers approve the research question and the proposed methods. The advantage of RR is that the emphasise is on the research question and rigour of the methods, rather than on the results. It protects research from practices that are aimed at “improving” the results.
The talk will describe the process of submitting and reviewing and RR, provide few examples, and highlight points to consider when preparing an RR. These will specifically concerns considering the study power, methods for evaluating the quality of the data and level of methodological detail required.
17:15 (Paris) / 11:15 (NYC)
Round Table
17:45 (Paris) / 11:45 (NYC)
Live posters & virtual socials
Tuesday 6 October – Track 1
Two parallel tracks – you can jump from one to the other
Time
Speaker’s name
Talk Link
Collaborative tools (Chair: Karim Jerbi)
14:00 (Paris) / 8:00 (NYC)
Guiomar Niso
BIDS: a data standard to support good scientific practices in neuroimaging
The Brain Imaging Data Structure (BIDS) is a community-led standard for organizing, describing and sharing neuroimaging data. Currently, it supports many neuroimaging modalities, such as MRI, MEG, EEG, iEEG and more to come. Multiple applications and tools have been released to make it easy for researchers to incorporate BIDS into their current workflows, maximising reproducibility, data sharing opportunities and supporting good scientific practices. This talk will share an overview of the BIDS current status and related tools to facilitate this endeavour.
14:20 (Paris) / 8:20 (NYC)
Martina G. Vilas
The Turing Way: A guide to reproducible, ethical and collaborative research practices
Reproducible research is necessary to ensure that scientific output can be trusted and built upon in future work. But conducting reproducible research requires skills in data management, software development, version control, and continuous integration techniques, that are usually not taught or expected of academic researchers.
The Turing Way is an open-source, community-led handbook that supports this knowledge in an accessible and comprehensible form for everyone. Its moonshot goal is to make reproducible research “too easy not to do”. In addition to discussing different approaches for reproducibility, it also provides material on ethical practices in data science, inclusive collaborative work, and effective communication and management of research projects. The handbook has so far been collaboratively built with the help of more than 175 people from different disciplines and career stages within data research, who have contributed to the project’s online repository (https://github.com/alan-turing-institute/the-turing-way).
This talk will give an overview of The Turing Way book, project, and community, and will show how you can get involved in its development.
14:40 (Paris) / 8:40 (NYC)
Break
14:50 (Paris) / 8:50 (NYC)
Antonio Schettino
Open Science Framework: One Service to Rule Them All
To improve the trustworthiness of research output in many disciplines, an increasing number of journals and funding agencies encourage or require sharing of data, materials, and analysis protocols associated with each publication. Consequently, researchers are turning to comprehensive services that facilitate collaborative workflow with colleagues and evaluators. One of the most popular is the Open Science Framework (OSF), a free online platform developed by the non-profit organization Center for Open Science. The OSF allows researchers to manage, document, and share all the products of their workflow, from the preregistration of the initial idea to the preprint of the final report. In this talk, I will show how the OSF helped me open up my research workflow, guide the audience through one of my public OSF projects, and discuss challenges and lessons learned during the process.
15:15 (Paris) / 9:15 (NYC)
Round Table
15:45 (Paris) / 9:45 (NYC)
Break
Reliability (Chair: Guiomar Niso)
16:00 (Paris) / 10:00 (NYC)
Bertille Somon
Open science for better AI
In the last ten years, publications on artificial intelligence (AI) have nearly doubled, opening promising avenue for an increased use of AI in our daily life. One key feature and challenge of AI is the generalizability of algorithms, notably for (inverse-)reinforcement learning or transfer learning (Arora and Doshi, 2019). Alike many AI research, these areas have a common root in neuroscience and psychology (Hassabis et al., 2020). Although working on freely available databases is becoming practice in computer science (e.g. ImageNet), it is not the case for neuroscience and psychology (except for the brain-computer interface community; e.g. BNCI Horizon 2020 project). Yet, a recent brain imaging study (Botvinik-Nezer et al., 2020) revealed the limits of reproducibility by sharing the same fMRI datasets with 70 research teams who analyzed them and identified consistent results for only 4 out of the 9 hypotheses tested. In the case of electroencephalography (EEG), generalizing data recording, processing and analysis is prevented from by: i) a lack of experimental design sharing; ii) recording format and parameters are not standardized (EEG systems have their own data format, compatibility and amplifier parameters); iii) processing pipelines are variable and not shared; iv) difficulties to store and share large datasets (Martinez-Cancino et al., 2020). We propose that following a general pipeline for setting-up experiments, collecting data, processing them and sharing files can increase the number of standardized datasets thus facilitating AI algorithms development for generalizability. This pipeline should be based on open access tools (LSL, BIDS, MNE, etc.). Sharing data allows AI researchers to improve modelling and machine learning algorithms quality, standardize algorithm performances comparison and allows laboratories with less means to perform quality research; but also it generalizes the possibility to get feedback from the whole community in an easier manner.
16:20 (Paris) / 10:20 (NYC)
Yuri Pavlov
#EEGManyLabs: Investigating the Replicability of Influential EEG Experiments
Since its discovery in the early 20th century, the recording of electrical brain activity, the electroencephalogram (EEG), from the scalp has had a profound impact on our understanding of human cognition. Today, EEG is a widely used tool to examine brain responses associated with cognitive functioning. Yet, despite its ubiquity, EEG research suffers from the same problems found across the human cognitive neurosciences, if not most of the behavioural sciences, of testing novel hypotheses in small samples with highly multidimensional data-sets that allow for practically unconstrained experimenter degrees of freedom. In the broader psychological sciences, a similar situation led many research groups to test the robustness of numerous influential findings via direct and systematic replications. These investigations revealed that a meaningful proportion of the psychological literature could not be replicated and highlighted the need for large subject samples to determine accurate and precise effect sizes. Inspired by these efforts and with a desire to examine the foundations of the field, we have launched #EEGManyLabs, a large-scale international collaborative effort investigating the reproducibility of EEG research, with an emphasis on studies involving event-related potentials (ERP). We have identified 20 highly cited studies in the literature and plan to directly replicate the key findings from each article across groups of at least three independent laboratories. Prior to data collection, the design and protocol of each replication effort will be subjected to peer review and, once the experiment is complete, each replication will be published as a Registered Report in Cortex. This work has the potential to provide evidence about the reproducibility of some of the most influential EEG studies, generate one of the largest open access EEG datasets to date, and promote the use of multi-lab collaborative efforts for cognitive neuroscience investigations.
16:40 (Paris) / 10:40 (NYC)
Break
16:50 (Paris) / 10:50 (NYC)
Aina Puce
Life after COBIDAS MEEG?
Magnetoencephalography [MEG] and electroencephalography [EEG] are neuroimaging methods that have a place in the rich history of the field we collectively refer to as non-invasive neurophysiology [here I refer to this as MEEG]. Recently, however, there has been renewed interest in these methods, resulting in many scientists and engineers from very different backgrounds entering the field and requiring training in both theory and methods. As our hardware technologies and software algorithms improve, this gives us new opportunities with which to study brain-behavior relationships. This requires evaluating and evolving our best practices so that we can perform robust and reproducible science. It is in this spirit that the Organization for Human Brain Mapping [OHBM] has become actively involved in promoting best practices in neuroimaging and in commissioning white papers and standards for best practices in different imaging modalities. It began with MRI-based methods (see COBIDAS MRI, Nichols et al., 2017) and continued with MEEG (see COBIDAS MEEG, Pernet et al., 2020). These white papers are living documents – to be updated as methods [new technologies, new analysis procedures] change in our field. Importantly, these documents have been, and will continue to be developed and revised with extensive input from the neuroimaging community. In today’s talk, I will discuss what future challenges we will have for COBIDAS MEEG V 2.0, what is likely to become obsolete and what new topics we will need to consider adding to improve the best practice guidelines for the future.
17:15 (Paris) / 11:15 (NYC)
Round Table
17:45 (Paris) / 11:45 (NYC)
Live posters & virtual socials
Tuesday 6 October – Track 2
Two parallel tracks – you can jump from one to the other
Time
Speaker’s name
Talk Link
Data Collection (Chair: Anne-Sophie Dubarry)
14:00 (Paris) / 8:00 (NYC)
John Mosher
Best Practices in Clinical MEG – Patient Preparation and Data Acquisition
We present best practices for the MEG recording for patients with epilepsy, from our years of experience in conducting over 3,000 patient exams at Cleveland Clinic and UT Houston. We emphasize the preparation of the patient and the setup of MEG instrument to ensure a quality clinical recording. Several practices are quite general for any MEG instrument, such as generally insisting on MRI compatible gowns, careful acquisition of landmarks on the patient’s scalp, and the daily recording of empty room data. The techniques address a gap in the clinical literature addressing the multitude of potential sources of error during patient preparation and data acquisition, and how to prevent, recognize, or correct those.
14:25 (Paris) / 8:25 (NYC)
Katia Lehongre
Collection of continuous and long term multiscale iEEG recordings
Patients with pharmaco-resistant focal epilepsy who are candidates for surgical treatment may need a presurgical evaluation with intracerebral electrodes to define the seizure-onset-zone. During this intracranial exploration, it is now possible to add microelectrodes without impact on the clinical investigation. Those microelectrode recordings give a rare access to single unit activity in humans. At the Pitié Salpétrière Hospital in Paris, we use microelectrodes since 2010 and collect and store long term and continuous recording of macro and micro electrodes simultaneously since 2012. The precious data acquired however also give rise to a data storage challenge, because of the high sampling rate of microelectrodes (32 kHz) and the large number of channels (up to 160) . During this presentation, I will describe our recording environment as well as the data management that has been setup, with its limits and possible improvements.
14:50 (Paris) / 8:50 (NYC)
Break
15:00 (Paris) / 9:00 (NYC)
Giovanni Mento
Concerns and joys of doing EEG/ERPs research across development
The recent application of neuroimaging techniques in the field of human cognitive development is providing the methodological basis for researchers to face speculative queries that were impossible to address before, enabling them to respectively describe “how the brain is” and “how the brain works” at birth or even before. In this sense, the use of electroencephalography has proved to be a reliable tool to depict how the brain process the environmental information (event-related activity) as well as how it is intrinsically organized at a functional level (resting-state activity). In this talk I will take a methodological overview on the use of EEG across developmental age, touching both age-specific issues (i.e., from birth to school-age) and analytic approaches (i.e., the use of brain source reconstruction).
15:25 (Paris) / 9:25 (NYC)
Emily Kappenmann
ERP CORE: An Open Resource for Human Event-Related Potential Research
Event-related potentials (ERPs) have broad applications across basic and clinical research, and yet there is little standardization of ERP paradigms and analysis protocols across studies. To address this, we created ERP CORE (Compendium of Open Resources and Experiments), a set of optimized paradigms, experiment control scripts, data processing pipelines, and sample data (N = 40 neurotypical young adults) for seven widely used ERP components: N170, mismatch negativity (MMN), N2pc, N400, P3, lateralized readiness potential (LRP), and error-related negativity (ERN). This resource makes it possible for researchers to 1) employ standardized ERP paradigms in their research, 2) apply carefully designed analysis pipelines and use a priori selected parameters for data processing, 3) rigorously assess the quality of their data, and 4) test new analytic techniques with standardized data from a wide range of paradigms.
15:50 (Paris) / 9:50 (NYC)
Round Table
16:20 (Paris) / 10:20 (NYC)
Break
Coded Tools (Chair: Andrea Brovelli)
16:35 (Paris) / 10:35 (NYC)
Marijn Van Vliet
Designing analysis code that scales
Small projects grow up to be large projects. That simple analysis script you started out with was so innocent, so uncomplicated, so pure. Where have those days gone? Staring at your code folder now is like staring into the Abyss…and it stares back! In this talk, we’ll be thinking about how to organize code in such a way that things scale as we add more analysis steps, collect more data, and explore more avenues. Complexity is our biggest enemy. It must be fought every minute of every day. Lose sight of this for only an instant and it will grow out of your control… We will use an EEG analysis pipeline as an example to see how we can compartmentalize complexity to contain the monster. I’m going to show you how to be faster, more accurate and generally more happy with your analysis code. Many of the ideas in this talk are outlined in the paper: https://doi.org/10.1371/journal.pcbi.1007358
16:50 (Paris) / 10:50 (NYC)
Laurens Krol
Simulating Event-Related EEG Activity using SEREEGA
With EEG, we try to measure at the scalp what happens inside the brain. It is difficult to evaluate how well our EEG analysis pipelines can estimate ground-truth brain activity, because no actual ground truth is available to compare the results to. Therefore, in order to test and evaluate EEG analysis methods, simulated data can be used instead. Because simulated data can be constructed such that the ground truth is precisely known. it can be used, among other things, to assess or compare the results of signal processing and machine learning algorithms, to model EEG variabilities, and to design source reconstruction methods. Previously, no general-purpose, standardised simulation toolbox was available: researchers generally implemented their own data simulation algorithms from scratch. Published in 2018, SEREEGA is a free and open-source MATLAB-based toolbox to simulate event-related EEG activity. It integrates with EEGLAB, is modular and extensible, and makes EEG data simulation easily accessible and reproducible. This talk will introduce the general philosophy of SEREEGA, as well as some basic simulation steps using both the graphical user interface, and the scripting language. See https://github.com/lrkrol/SEREEGA for more information about the toolbox.
17:05 (Paris) / 11:05 (NYC)
Break
17:15 (Paris) / 11:15 (NYC)
Etienne Combrisson
Framework for Information Theoretical analysis of Electrophysiological data and Statistics (FRITES)
We will present the computational framework and neuroinformatics tools for assessing the statistical significance of information-theorical measures computed on neurophysiological data, such as MEG and intracranial SEEG signals. The framework and tools allow the quantification of task-related modulations in the activity of single brain areas (e.g., source-level MEG high-gamma activity) and from functional connectivity (FC) measures computed on pairs of brain regions (e.g., Granger causality). Group-level inference is performed by combining cluster-based methods with either fixed-effect or random-effect approaches. We will also present the toolbox and github repository.
17:30 (Paris) / 11:30 (NYC)
Round Table
17:45 (Paris) / 11:45 (NYC)
Live posters & virtual socials
Wednesday 7 October
Time
Speaker’s name
Talk Link
Software (mis)Uses (Chair: Deirdre Bolger)
14:00 (Paris) / 8:00 (NYC)
Alex Gramfort
What to do and **not** to do with MNE-Python?
Magnetoencephalography and electroencephalography (M/EEG) measure the weak electromagnetic signals induced by brain electrical activity. Using these signals to characterize and locate brain activations is a challenging task as confirmed by three decades of methodological contributions. MNE, which holds its name from its historical ability to compute minimum norm estimates, is a
software package that addresses this particular challenge by providing a state-of-the-art analysis workflow spanning preprocessing, various source localization methods, statistical analysis, and estimation of functional connectivity between distributed brain regions. This talk aims to present good practices when working in Python and in particular using MNE. I will mention suggestions for development environments, how to efficiently organize, profile or debug your code etc. Full documentation, including many
examples and tutorials, is available at https://mne.tools/.
14:15 (Paris) / 8:15 (NYC)
Scott Makeig
Implementing your own best practice using EEGLAB
Leading (or cutting) edge research has two interconnected aspects. The first is exploratory — using new measures to open and look through new windows into natural phenomena never before seen or appreciated. The second is confirmatory — testing hypotheses generated by combining results of previous confirmatory and exploratory research with scientific reasoning and imagination. Arnaud Delorme and I created EEGLAB to serve both these purposes. Currently, alarm over use of weak statistical reasoning in neuroimaging is promoting robust confirmation practices, but this should not also deter imaging researchers from exploring their data. The legacy of methods historically used to reduce MEEG data to a few measures has failed to capture much of its rich information about brain dynamics supporting experience and behavior. The EEGLAB processing environment and its more than 100 plug-in packages makes readily available a wide range of new and old data measures and visualization methods, plus the robust LIMO statistical framework for hypothesis testing. Current advances in EEGLAB include BIDS format conversion with HED event annotation, direct connectivity to the XSEDE high-performance computing network via the Neuroscience Gateway, and high-resolution MEEG source localization and SCORE-optimized head modeling.
14:30 (Paris) / 8:30 (NYC)
Break
14:40 (Paris) / 8:40 (NYC)
Sylvain Baillet
How good software enables good scientific practices.
Good scientific practices are glorified, for obvious good reasons, but can be simply impractical to us mortals. Scientific software is key to enable the adoption and adherence to righteous practices in practice. I will show features available in Brainstorm that aim to facilitating everyone’s virtuous data management and data analytics life: from data organization with BIDS, to building pipelines that are shareable and reproducible.
14:55 (Paris) / 8:55 (NYC)
Robert Oostenveld
How FieldTrip can help you with good scientific practices
Using the right tools and methods is crucial to get the most out of your MEG and EEG data, and obtaining good results requires that you understand how to optimally use these analysis methods. However, disseminating or sharing your results involves more than just using these analysis methods and reporting on their outcomes in a paper. There is much more knowledge that you are acquiring during your study, for example what the best analysis strategy is, or the most optimal settings of that specific algorithm. I will present recent advancements in the FieldTrip toolbox that support you in managing and sharing your data using BIDS, and in sharing the details of your analysis pipeline. Both your primary findings (i.e. your publication), your data, and your insights on how to best analyze the data are crucial to bring the field forward and to create the biggest impact of your research!
15:10 (Paris) / 9:10 (NYC)
Round Table
15:40 (Paris) / 9:40 (NYC)
Break
Signal processing (Chair: Christian Bénar)
15:55 (Paris) / 9:55 (NYC)
Karim Jerbi
MEG and EEG in the age of machine learning: Data-driven versus Hypothesis-driven science
The recent proliferation of studies using brain decoding and data-driven methods has brought a lot of interest and excitement to the field of MEG and EEG. Amid strong claims about the endless power of machine learning (ML) techniques, including data representation learning, legitimate questions arise: Is the age of good-old hypothesis-driven neuroscience coming to an end ? What is the added value of ML for MEG/EEG research ? What are the good practices and pitfalls of ML analytics that we need to be aware of? And finally, how will I be able to discuss all this in twenty minutes ?
16:20 (Paris) / 10:20 (NYC)
Jean-Marc Lina
Good practice in time-frequency analyses
“Time-frequency analyses are frequently used in biomedical signal processing. This presentation will first explore the natural path from the time series to the wavelet decomposition of rhythmic signals, passing through the basic steps: the band pass filtering and Hilbert transform, the Morlet and analytic wavelet transform. In the second part, the discrete wavelet decomposition will be discussed in the study and characterization of the concomitant arrhythmicity of electrophysiological signals .”
16:45 (Paris) / 10:45 (NYC)
Break
16:55 (Paris) / 10:55 (NYC)
Alex Gramfort
Are you asking too much to your MEG/EEG source imaging results?
If the number of parameters to estimate exceeds the number of measurements, an estimation problem is said to be ill-posed. Due to limited acquisition times, physics of the problems and complexity of the brain, the field of brain imaging needs to address many ill-posed problems. Among such problems is the localization in space and time of active brain regions with magneto-encephalography (MEG) and electro-encephalography (EEG). Some people will tell you that the problem of M/EEG source imaging is just too hard to be reliable for scientific findings. In this talk I will try to discuss this statement and present methodological strategies to evaluate if you can be confident that your activation maps can be trusted.
17:20 (Paris) / 11:20 (NYC)
Round Table
17:45 (Paris) / 11:45 (NYC)
Live posters & virtual socials
Thursday 8 October
Time
Speaker’s name
Talk Link
Statistics (Chair: Guillaume Rousselet)
14:00 (Paris) / 8:00 (NYC)
Maximilien Chaumon
Statistical power in MEG data: spatial constraints for a reliable study
Statistical power is key to robust, replicable science. Here I will first remind everyone of the definition of statistical power, why it is important in data analysis, and in particular in MEEG. I will then highlight what increasing power often boils down to when planning an experiment, namely increasing trials and subjects number. We recently manipulated these variables in simulated experiments to find out the ideal number of trials and subjects. The answer is – as often – more complex than the question, and a step back is required here because many parameters come into play. In this study, we focus on a specific set of parameters: the spatial properties of the expected neural sources. I will illustrate the well-known effects of distance and orientation, as well as the less well documented effects of inter-subject variability on statistical power. Finally, I report on the effect of simple and widely used manipulations such as squaring the data before testing on statistical power. Generally, I want to advocate here for critical scrutiny and attention to expected sources of variability while planning an M/EEG experiment.
14:20 (Paris) / 8:20 (NYC)
Aaron Caldwell
Using the Superpower R package to simulate power
Power analysis has become the sine qua non for justifying for sample sizes in experimental studies. For simple one or two sample comparisons, the process is fairly straightforward. When the experiments become more complex, such as when factorial designs are implemented, the tools used for power analysis are sparse and the calculations become more difficult. A simple solution to the problem of design complexity is just to simulate the study design in order to estimate power. However, simulation tends to require more technical knowledge and some ability to write code. Therefore, Superpower R package was created to streamline the simulation process and make simulation tools accessible for the average researcher. Currently, the package allows for Monte Carlo and “exact” simulations for factorial designs with both within and between subjects factors. This allows for power estimates for ANOVA, MANOVA, and estimated marginal means comparisons. In addition, this is a useful teaching tool as it can show how violating assumptions (e.g., homoskedasticity or sphericity) can affect both statistical power and type 1 error rates. In this presentation, I will demonstrate 1) why these principles are important 2) how Superpower, in both its R and Shiny formats, can be useful and 3) how non-simulation based functions can be used to justify your alpha level.
14:40 (Paris) / 8:40 (NYC)
Break
14:50 (Paris) / 8:50 (NYC)
Steven Luck
Standardized measurement error: A universal measure of data quality for averaged event-related potentials
Event-related potentials (ERPs) can be very noisy, and yet there is no widely accepted metric of ERP data quality. Here we present a universal measure of data quality for averaged ERPs: the standardized measurement error (SME). Whereas some potential measures of data quality provide a generic quantification of the noise level, the SME quantifies the expected error in the specific amplitude or latency value being measured in a given study (e.g., the peak latency of the P3 wave). It can be applied to virtually any value that is derived from averaged ERP waveforms, making it a universal measure of data quality. In addition, the SME quantifies the data quality for each individual participant, making it possible to identify participants with low-quality data and “bad” channels. When appropriately aggregated across individuals, SME values can be used to quantify the impact of the single-trial EEG variability and the number of trials being averaged together on the effect size and statistical power in a given experiment. If SME values were regularly included in published papers, researchers could identify the recording and analysis procedures that produce the highest data quality, which could ultimately lead to increased effect sizes and greater replicability across the field. The SME can be easily calculated using the latest version of ERPLAB Toolbox (v8.0).
15:10 (Paris) / 9:10 (NYC)
Round Table
15:30 (Paris) / 9:30 (NYC)
Break
Guest session: ARTEM-IS:
Agreed Reporting Template for EEG Methodology – International Standard
(Chair: Anđela Šoškić)
16:10 (Paris) / 10:10 (NYC)
Anđela Šoškić
Does reporting of ERP methods support replicable research? The current state of publication for N400s and beyond
For decades, researchers in the fields of EEG and ERP have made calls for better transparency in the arena of reporting EEG/ERP data (e.g. Donchin et al., 1977, Picton et al., 2000; Keil et al., 2014; Duncan et al., 2009; Kappenman & Luck, 2016, Taylor & Baldeweg, 2002; Luck, 2014; Luck & Gaspelin, 2017; Gelman & Loken, 2013). Despite the availability of guidelines for transparent and accurate reporting, the state of the published literature suggests that authors are either unable or unwilling to follow current reporting guidelines. We propose that by leveraging the collective expertise of stakeholders in the ERP community, we can create an agreed reporting framework that is both easier to use and more transparent than current reporting models.
Background. One recent systematic review (Šoškić, Jovanović, Styles, Kappenman & Ković, 2019: Preprint https://psyarxiv.com/jp6wy/) has demonstrated that published journal articles rarely contain all of the information that would be required to replicate an N400 study, and that the reporting was inconsistent and often ambiguous, making it hard to critically assess, metaanalyse and replicate work. This project and its sister projects (Šoškić, Kappenman, Styles & Ković, Preregistration 2019: https://osf.io/6qbjs/; Ke, Kovic, Šoškić, & Styles, Preregistration, 2020: osf.io/5evc8) show that improvements to reporting standards in the ERP field will be necessary to increase replicability and reduce questionable research practices.
Current Presentation. On the basis of our metanalytic work, we are drafting a metadata template (i.e., a digital form or template to be filled), containing all of the metadata critical to an ERP recording and the subsequent processing and analysis chain. In contrast to the valuable work of Keil et al. (2014) and Pernet et al. (2018), who developed checklists for authors to indicate whether they have reported appropriate methodology details in the body of their article, the metadata template requires precise numerical/categorical data to be filled, thereby ensuring searchability and simplicity in future metascience. To facilitate transparency in this process, we want to engage the community of stakeholders in EEG/ERP research, in a consultative process to refine the template, and move towards an agreed reporting framework – we envisage a consultative process that involves frontline researchers, software developers, data archivists, journal editors and researchers with experience in open, replicable science.
16:30 (Paris) / 10:30 (NYC)
Suzy Styles
How can a community of skilled practitioners leverage their collective expertise to improve standards? Lessons from aviation and surgery
TBA
16:45 (Paris) / 10:45 (NYC)
Break
16:55 (Paris) / 10:55 (NYC)
Round Table – Emily Kappenman, Bertille Somon, Giorgio Ganis
17:45 (Paris) / 11:45 (NYC)
Live posters & virtual socials
Friday 9 October
Time
Speaker’s name
Talk Link
Beyond The Signal (Chair: Manuel Mercier)
14:00 (Paris) / 8:00 (NYC)
Daniele Schon
Changing scales for a changing science: philosophical, sociological and ethical considerations
In the last decades we have assisted to an impressive increase of numbers across most scientific communities. More electrodes, more participants, more data, more methods, more publications, more scientists. To this extent the scales of science are changing. But how do they evolve? What are the factors that exert selection pressure? And what is the added value?
I will possibly give two short examples from art and literature to show that large scales and large numbers are not necessarily more informative than small scales and small numbers. I will then show some quantitative analyses of how long it takes us to publish, how much we publish, how science communication media change, how many people we are, how much science costs, how much we pollute etc…
While I am not an expert in the field, I will be happy to share with an informed scientific audience my modest knowledge and the many questions that emerge from such a reflection. Here is one, quoting Marcel Proust: “The real voyage of discovery consists, not in seeking new methods, but in having new eyes” (the word in italic is mine, the original is “landscape”).
14:25 (Paris) / 8:25 (NYC)
Yseult Héjja Brichard
Rethinking our Narratives: The Challenge of “Slow Science”
In response to a replication crisis over the last decade, the Open Science movement has attempted to promote different tools and research practices. New incentives and platforms were designed to improve the replicability of studies and to address different biases, such as the positive result bias. For many, this is the new golden path to follow if we want our scientific productions to be trustworthy. However, by offering concrete solutions to problems so-far identified by the scientific community, the open science movement might only cover the superficial layer of a deeper malfunction of our research practices and structures. In this talk, I propose to review the main concepts of the slow science philosophy to discuss why and how we need to rethink our research frameworks, both inside and outside academia. More importantly, the slow science philosophy also questions what it means to do science and to be a researcher and what is or should be the position of a researcher in our societies.
14:50 (Paris) / 8:50 (NYC)
Break
15:00 (Paris) / 9:00 (NYC)
David Poeppel
Spatial resolution is great. Temporal resolution is greater. “Conceptual resolution” is best.
TBA
15:30 (Paris) / 9:30 (NYC)
Round Table
15:50 (Paris) / 9:50 (NYC)
Closing Remarks
How To
Attending the event
LiveMEEG is an online conference using the crowdcast platform. Registration is free but compulsory to participate fully. Instructions for registration will be provided shortly, stay tuned.
Poster submission
Submit your poster here. The deadline for submission is Sept. 23rd. Poster acceptance will be communicated shortly before the conference.
Social events
The posters will be displayed during social events open to registered participants.
Special Issue Warm-up
We have an in principle agreement of NeuroImage editors to create a special issue on “Advances in Scientific Practices”. The whys and wherefores of this special issue, as well as potential contributions will be discussed during the conference.
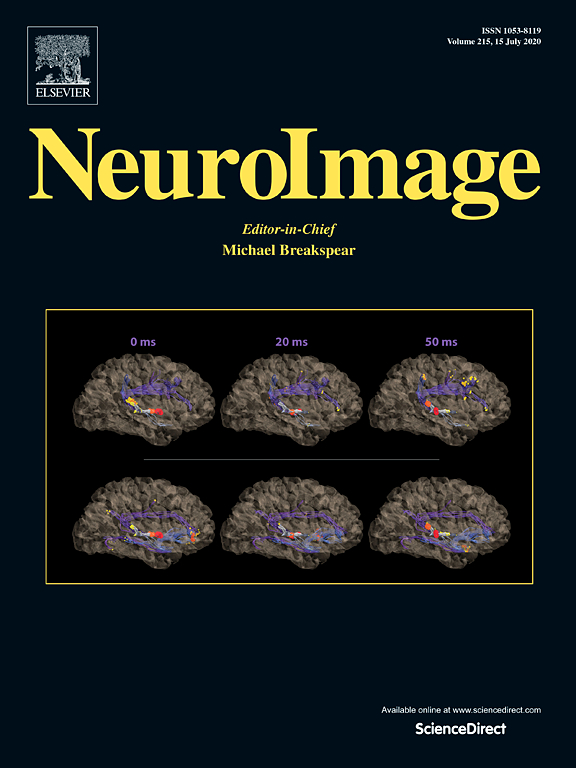
Organizing team
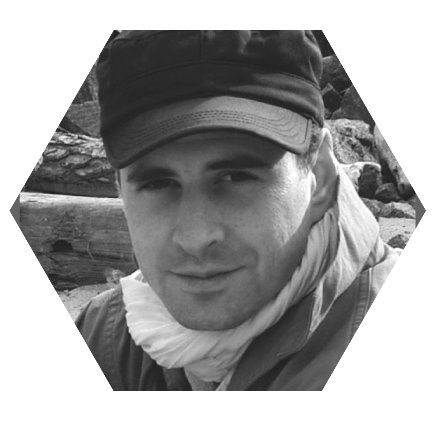
Manuel Mercier
INS, Inserm, AMU (France)
Our Academic Partners
Even an online event has important costs, we warmly thanks our partners for their support